Barriers to implementation of dynamic reference points in fisheries management
Abstract
Fish populations are dynamic; their productivity depends on the environment, predator and prey interactions, and fisheries harvest rates. Failure to account for these factors in fisheries science and management can lead to a misestimation of stock dynamics and productivity, resulting in overexploitation or forgone fisheries yield. Using an online survey, we asked fisheries scientists, industry stakeholders, Indigenous partners, and non-governmental organizations whether changing ecosystem productivity was a problem in their experience, how often dynamic approaches to fisheries reference points have been adopted, what methods had been used, and what fisheries they had been applied to. Changing fisheries or ecosystem productivity was reported as an issue by 96% of respondents; however, 74% of respondents said they had never seen dynamic reference points implemented, 16% said in very few instances, while 10% said frequently. The most common barriers to implementation of dynamic approaches in fisheries management were institutional inertia and uncertainty about whether a change in productivity was lasting. We discuss trade-offs between fisheries management performance and stability.
Introduction
Fisheries management generally aims to balance economic, social, and ecological goals—the relative importance of each varies according to culture, nation, and ecosystem type (Worm et al. 2009). Traditionally, fisheries have been managed at the species level (Hilborn and Walters 1992). Acknowledgement that environmental conditions, habitat, and food web interactions change through time and affect fished species has led toward managing at the ecosystem scale, referred to as ecosystem-based fisheries management (EBFM) (Fulton 2021; Howell et al. 2021; Link and Marshak 2021). In some cases, it has been speculated that collapse of fish stocks could have been prevented if EBFM had been in place (Lindegren et al. 2009). From a scientific perspective, challenges with implementing EBFM in dynamic systems lie in the detection of changes in the system and prediction of its future state (Travis et al. 2014; Dempsey et al. 2018). From a management perspective, challenges lie in how to respond to dynamic systems in a timely fashion to achieve management goals and to balance management performance and stability (Townsend et al. 2019; Collie et al. 2021; Kasperski et al. 2021; Spence et al. 2021).
Reference points are tools to quantify management objectives, which often include target and limit reference points of resource biomass and associated fishing pressure (Caddy and Mahon 1995). Reference points help to define fisheries sustainability by balancing fisheries yield, sustainability, and conservation objectives (Carpenter et al. 2017). Fisheries stock assessments evaluate whether fish stock biomass has increased or decreased from previous assessments based on fisheries catch data, fisheries-independent survey data (when available), and ecosystem data (when available). Unfished biomass (B0) is a commonly used reference point, often with a target to maintain fish stock biomass at 40%–60% of B0 and with a limit reference point to maintain biomass above 20%–30% B0. Biomass at maximum sustainable yield (BMSY)—the biomass level that maximizes long-term stock productivity, typically about 50% B0—has also traditionally been used as a target reference point (Hilborn and Walters 1992; Worm et al. 2009). Associated with target reference points are the corresponding fisheries exploitation rates (u) to achieve them, commonly represented as fisheries mortality (F) in population dynamics models (Hilborn and Walters 1992). Estimating biomass reference points for species with highly variable abundance and recruitment dynamics provides a challenge, and choice of reference point estimation method should consider species life history (Haltuch et al. 2009).
Fish stocks naturally fluctuate through time; of concern for setting reference points are trends in abundance and regime shifts (Klaer et al. 2015). Traditional practice derives reference points from equilibrium assumptions of population dynamics that are constant through time. However, there is increasing evidence that population dynamics can exhibit multiple equilibrium states, especially in response to climate change and ecosystem regime shifts (Szuwalski and Hollowed 2016; Zhang et al. 2018; Tang et al. 2021), or that they are cyclical or dynamic through time (Bakun et al. 2010). Traditional static reference points that assume stationary population dynamics can change over time when recalculated with updated data about vital rates (Berger 2019). Allowing reference points to vary through time to account for shifts in ecosystem and species population productivity may be necessary to realize fishery sustainability (Szuwalski and Hollowed 2016). We refer to the process of allowing changes in reference points over time as dynamic reference points.
Dynamic reference points differ from static reference points in the ability to incorporate non-stationary population dynamics and track time-varying population vital rates (e.g., population productivity; Berger 2019). Dynamic reference points can be implemented by either accounting for dynamic unfished biomass (B0) or by using dynamic fisheries exploitation rates (u and F) (Punt et al. 2014; King et al. 2015). Dynamic B0 is calculated by running a model without fisheries catch removals after population dynamics and fisheries mortality parameters have been estimated, making the crucial assumption that recruitment deviations and other model parameters are not density-dependent (Berger 2019). Dynamic B0 can also be estimated using time-varying parameter estimates from a stock assessment (e.g., recruitment deviations and natural mortality; MacCall et al. 1985). Dynamic F can be set based on an understanding of the stock productivity, which is influenced by environmental conditions, ecosystem productivity, and predator–prey interactions (Howell et al. 2021). Recent advances in state-space modelling for fisheries stock assessment provide an approach to estimate key time-varying population rates (e.g., Peterman et al. 2000; Peterman et al. 2003; Minto et al. 2013; Pedersen and Berg 2017; Holt and Michielsens 2020; Zhang et al. 2020), making it possible to generate dynamic reference points that track changes in population productivity (Collie et al. 2021). Other methods define recent population state based on a truncated time-series of recruitment (i.e., moving window, the sequential t test analysis of regime shifts (STARS) (Rodionov 2004; Rodionov and Overland 2005), with the trade-off that truncating a time-series introduces additional uncertainty and removes information from the analysis (van Deurs et al. 2021). Ecosystem model simulations can be used to produce ecosystem fishing morality values (Feco) to keep fishing intensities within predetermined ecosystem indicator limits (Howell et al. 2021). Finally, dynamic reference points have been created using covariates that define prevailing environmental conditions—although situations where environmental mechanisms are known are rare (i.e., Punt et al. 2014; Hill et al. 2019). Despite the existence of these methods, the practical application of dynamic reference points in fisheries management remains a challenge.
While there have been advances in approaches to calculate dynamic reference points, they may not always perform as well as static reference points (A’mar et al. 2009; Haltuch et al. 2009; Haltuch and Punt 2011; Punt et al. 2014; Berger 2019; O’Leary et al. 2020; Punt et al. 2021). Dynamic reference points typically outperform static reference points when there is a directional change in fish stock productivity, while static reference points are better suited to non-directional variability in population dynamics (Berger 2019). For example, west coast U.S. groundfish stock status indicators mostly varied by less than 10% when calculated with static vs. dynamic B0 approaches; however, in some cases status differed by as much as 72%, which would trigger different management actions (Berger 2019). Detecting a regime shift to trigger a change in reference point can be problematic, and attributing the mechanism of regime shift—abundance or spawning biomass driven vs. environment driven—is often difficult but important to inform the type of management action to be taken (Szuwalski 2013; Vert-pre et al. 2013a, 2013b; Punt et al. 2014; Klaer et al. 2015).
Here, we address whether dynamic reference points should be used, how and where they have been applied, and why they are not more widely used in fisheries management. To this end, we designed an online survey to ask fisheries scientists, industry stakeholders, Indigenous partners, and non-governmental organizations (NGOs) whether changing ecosystem productivity was a problem in their experience and how often dynamic approaches to fisheries reference points have been employed. We describe respondent background and experience, fisheries where dynamic reference points have been used, philosophy for their use, and methods to implement them. We also report the barriers to implementation of dynamic approaches in fisheries management. The aims of this study are to gain insight into how often dynamic reference points are implemented, the methods employed to do so, and explore reasons why they may not be used in situations where they could be useful.
Methods
We solicited experts and stakeholders that were invited to attend an online workshop on “Fisheries Management Reference Points in Highly Dynamic Ecosystems” from 25–29 January 2021 (Zhang et al. 2021) to participate in an online survey. The purpose of the workshop was to provide a general overview of the theory and implementation of dynamic reference points to inform fisheries management. We allowed snowball sampling by encouraging invitees to invite additional experts and stakeholders to respond between January 15, 2021 – March 31, 2021 (purposive sampling; Penrod et al. 2003). The purpose of the survey was to gain insight about respondent experience with dynamic reference points, fisheries where they had been implemented, methods used, and barriers to their implementation. We targeted individuals working in the field of dynamic reference points, although we acknowledge that not every expert was surveyed. Among respondents, we had good global coverage for countries that utilize dynamic reference points and consider our results generally representative; however, as with any sampling methodology, biases exist. Due to the location of the workshop and its hosts being based in Canada, we received more responses from Canadians, potentially overemphasizing a Canadian dynamic reference point perspective. While lack of internet access could prevent respondents from being able to participate in an online survey, we assumed that most active fisheries scientists providing reference points had access.
The survey had 18 questions about participant professional background, experience with dynamic reference points, philosophy on dynamic reference points, implementation of dynamic reference points, and methods to implement dynamic reference points (see Table 1 for complete list of questions). We received approval to conduct this survey from the Interdisciplinary Committee on Ethics in Human Research, Memorial University (ICEHR #20211291-MI). Not all questions were answered by all respondents (i.e., respondents who had no experience providing reference points did not answer questions that asked for details about which stocks and methods they had experience with).
Table 1.
Background |
1. Province/State, country of residence (withheld for anonymity) 2. Sector (gov’t, university, industry, NGO, other) (see Fig. 1) 3. Does your work involve providing reference points? 62% yes (n = 50), 38% no (n = 30) 4. Number of years experience providing reference points (see Fig. 1) 5. Country/countries where reference points have been provided (see Fig. 2). 6. Fisheries where reference points have been provided (see Fig. 3) |
Philosophy on Dynamic Reference Points |
7. Is changing ecosystem or fisheries productivity an issue in your experience? 93% yes, 7% no (n = 41) 8. Should reference points be changed each time an assessment is updated? 23 no = 29%, 19 yes = 24%, 3 unsure = 4%, 34 conditional = 43% (n = 79) 9. What is/are the criterion/criteria to change management reference points? • Change of ecosystem/fisheries productivity—n = 65 • Change of population dynamics estimated by stock assessment models—n = 47 • Change of biological traits (e.g., growth rate and maturation)—n = 53 • Other (please specify) 10. Should reference points change, how often, and if so, what do you think would be the best process that leads to change? (see Fig. 4) |
Implementation of Dynamic Reference Points |
11. How often do you see implementation of dynamic reference points in management practices? • Never—n = 38 (74%) • Very few—n = 8 (16%) • Frequently—n = 5 (10%) 12. If you have seen implementation of dynamic reference points, for which fisheries are they applied? • Lobster • Herring • Capelin • Atlantic cod • Menhaden • Harp and grey seals • Pacific salmon • Snow crab • Scallop • Groundfish 13. If you think dynamic reference points are important, but not yet implemented, why aren’t they? (see Fig. 5) |
Methods to Implement Dynamic Reference Points |
14. Please identify method(s) to address dynamic reference points that you are familiar with (including references) (see Fig. 6) 15. Has/have this/these method(s) been applied? If so, please provide examples • Spawning biomass per recruit (SBPR) for crab and groundfish • Dynamic B0 for scallop • Variable M for cod • Dynamic B0 for tuna • Double hockey stick for cod and menhaden • Dynamic B0 for scallops • Dynamic B0 for harp and grey seal 16. Where are these methods useful? • Strong understanding of system dynamics • Strong shifts in productivity • Data rich systems • Where climate drivers are important • Assessments • Double hockey stick for stocks at high biomass levels • Feco useful wherever there is a desire to account for ecosystem factors that are not directly implementable in single species simulations • Multispecies harvest control rules should really be the norm but run into political differences in terms of trade-offs between fleets • Where keystone species affect dynamics • Data-limited system • Changes in fishery 17. Where are these methods limited? • Data-limited system, n = 6 • Uncertainty about change in productivity, n = 6 • Uncertainty about system dynamics, n = 6 • When fishing alters biological parameters, n = 1 18. Data situation where this/these method(s) is/are applicable or not • Data rich, n = 18 • Able to identify changes in productivity, n = 3 • Changes in productivity are not due to fishing, n = |
As above, there are different ways that dynamic reference points can be implemented—by accounting for variable B0 or by updating static B0. For the purposes of the survey, we did not differentiate between the two approaches; however, respondents were asked to provide methods to implement dynamic reference points. We explored whether respondent background and fisheries that respondents had provided reference points for explained variance in survey responses.
Results
Participant background
We received survey responses from 82 participants across government, Indigenous partners, industry, intergovernmental organizations (i.e., International Council for the Exploration of the Sea (ICES)), non-governmental organizations, and universities, who ranged from 0 to 20 years experience providing reference points (Fig. 1). Participants who had the most experience providing reference points were from intergovernmental organizations (mean = 12.5 years; standard deviation (SD) = 10.6), industry (mean = 8 years; SD = 7.6), and government (mean = 5.4 years; SD = 3.8), while some participants from universities also had high experience (mean = 5.2 years; SD = 7.0; Fig. 1). Participants had experience providing reference points in 51 countries, with most of the experience in Canada, the US, and Europe (Fig. 2). Survey respondents had experience providing reference points for 29 fishery types, of which Atlantic cod (Gadus morhua) and snow crab (Chionoecetes opilio) were the most frequent, followed by shrimp, demersal fish, Pacific salmon (Oncorhynchus spp.), and redfish (Sebastes fasciatus; Fig. 3).
Fig. 1.
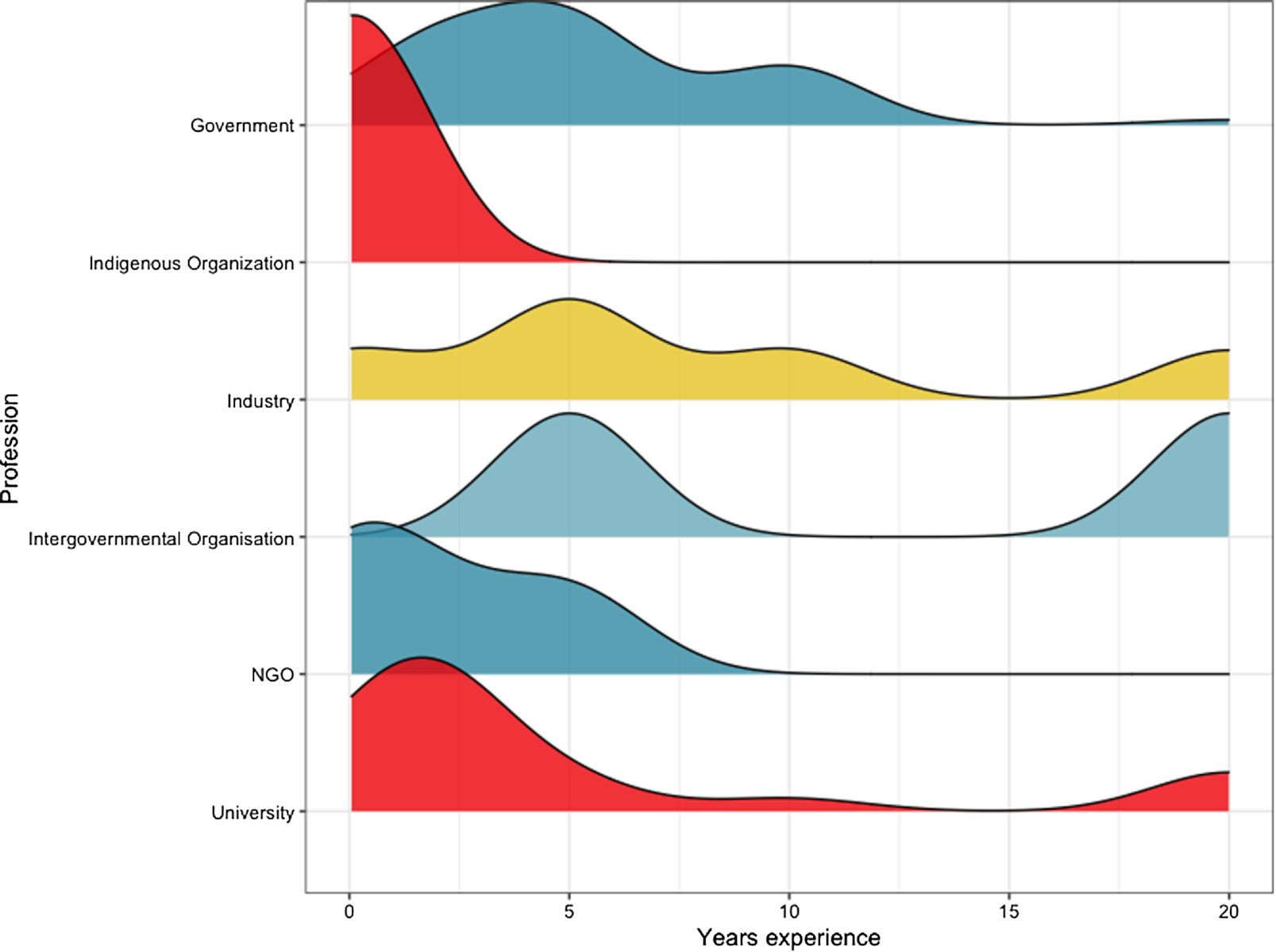
Fig. 2.
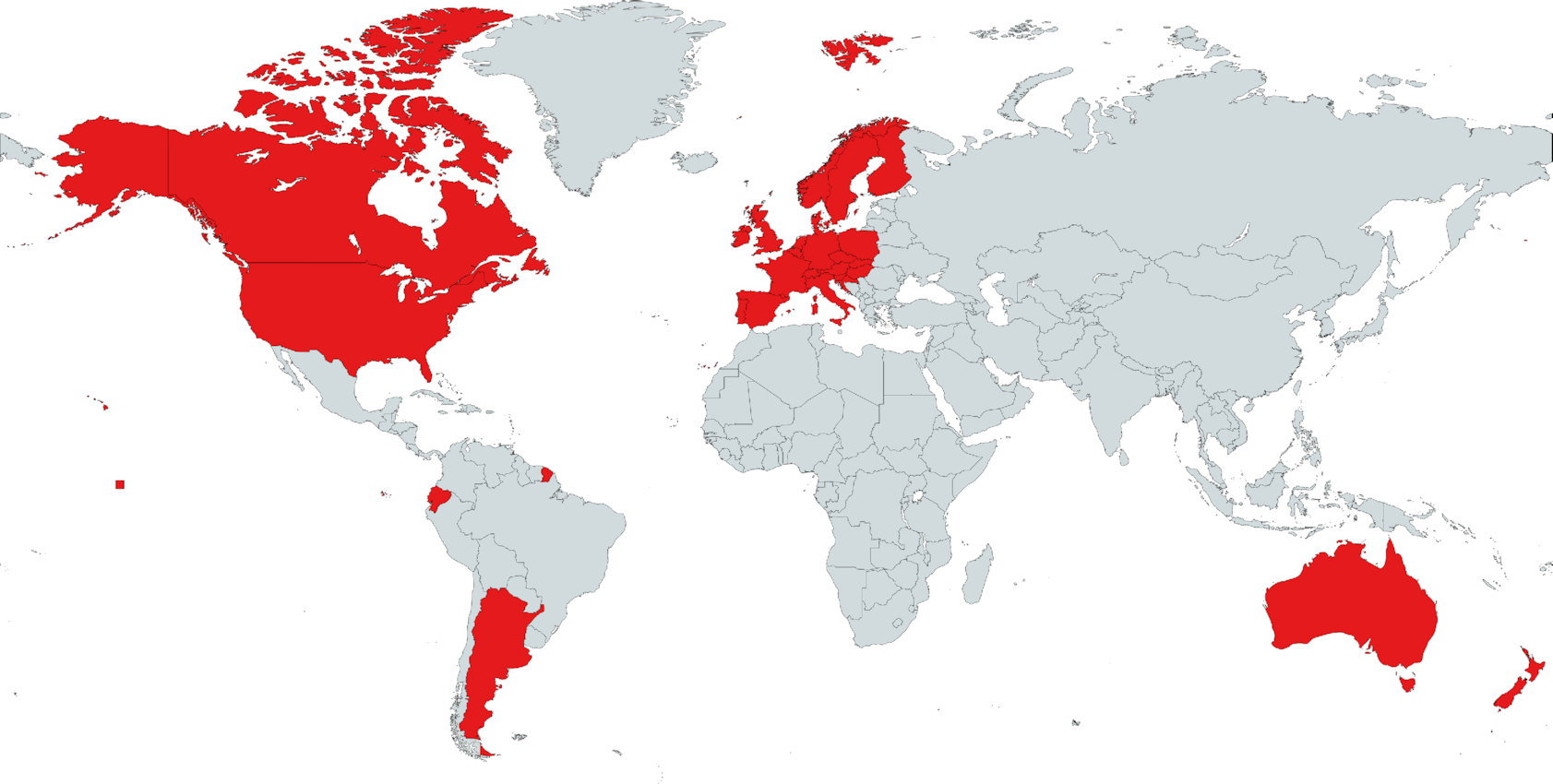
Fig. 3.
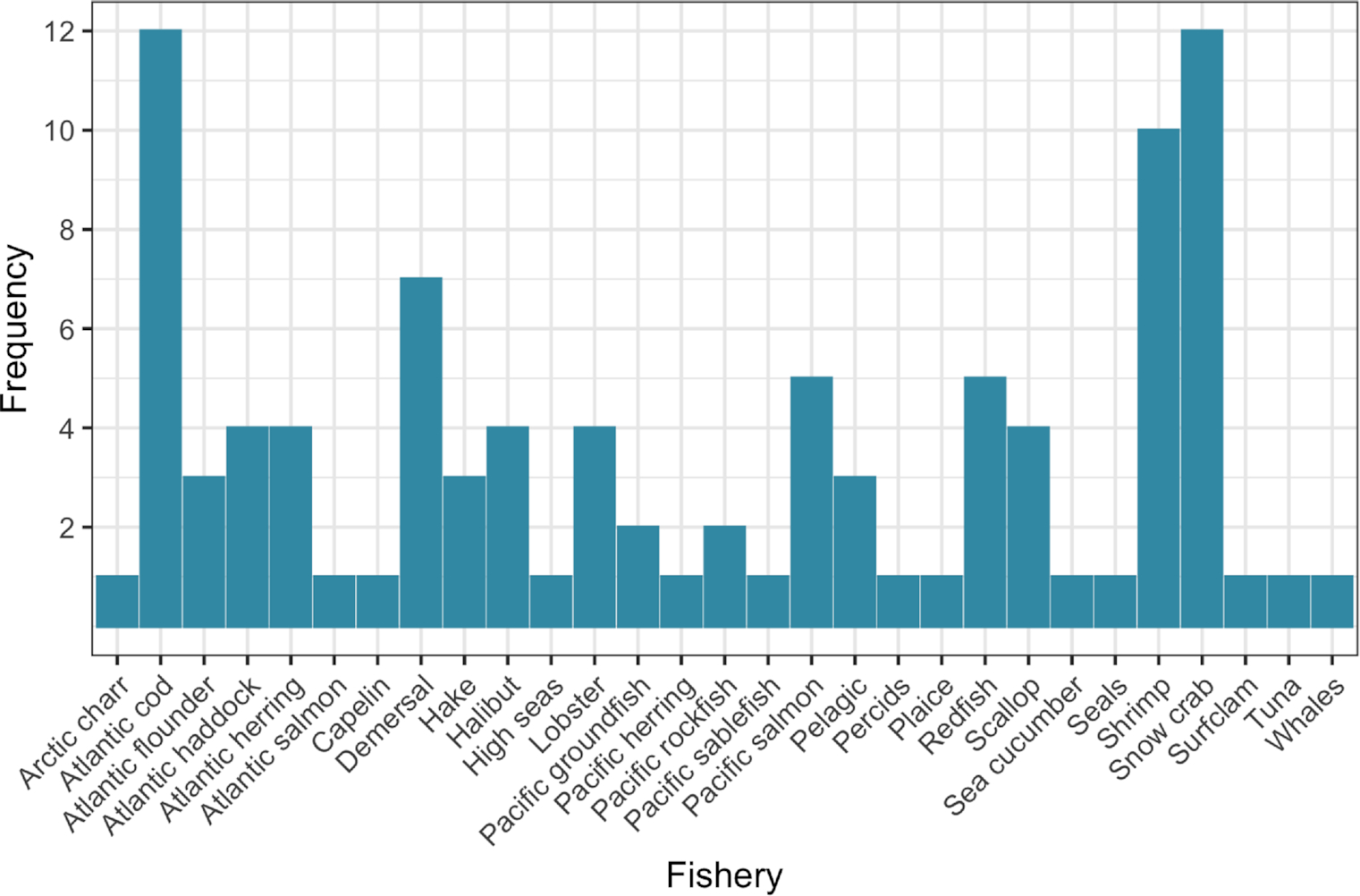
Philosophy on dynamic reference points
When asked whether changing ecosystem or fisheries productivity had been an issue for reference points, most respondents indicated yes (96%; n = 77). Experience providing reference points was not a major factor explaining these responses, as 96% of respondents who had provided reference points indicated that changing ecosystem or fisheries productivity had been an issue in their experience (n = 48). 24% of respondents (n = 19) thought reference points should be changed each time an assessment was updated, 29% (n = 23) thought they shouldn’t, 43% (n = 34) said it was conditional, while 4% (n = 3) were unsure. We explored whether the fisheries respondents had experience with explained variability in these responses, but there were no clear trends. When asked what is/are the criterion/criteria to change management reference points, 65 respondents indicated when there was a change in ecosystem or fisheries productivity, 53 respondents said when there was a change in biological traits, and 47 respondents said when there was a change in population dynamics estimated by stock assessment models. When asked when or under what circumstances reference points should change, the most popular answer was with changes in the ecosystem (n = 23), followed by with changes in the environment (n = 8), followed by every 5 years (n = 5), every 5–10 years (n = 3), with each assessment (n = 2), and every 3–5 years (n = 1; Fig. 4).
Fig. 4.
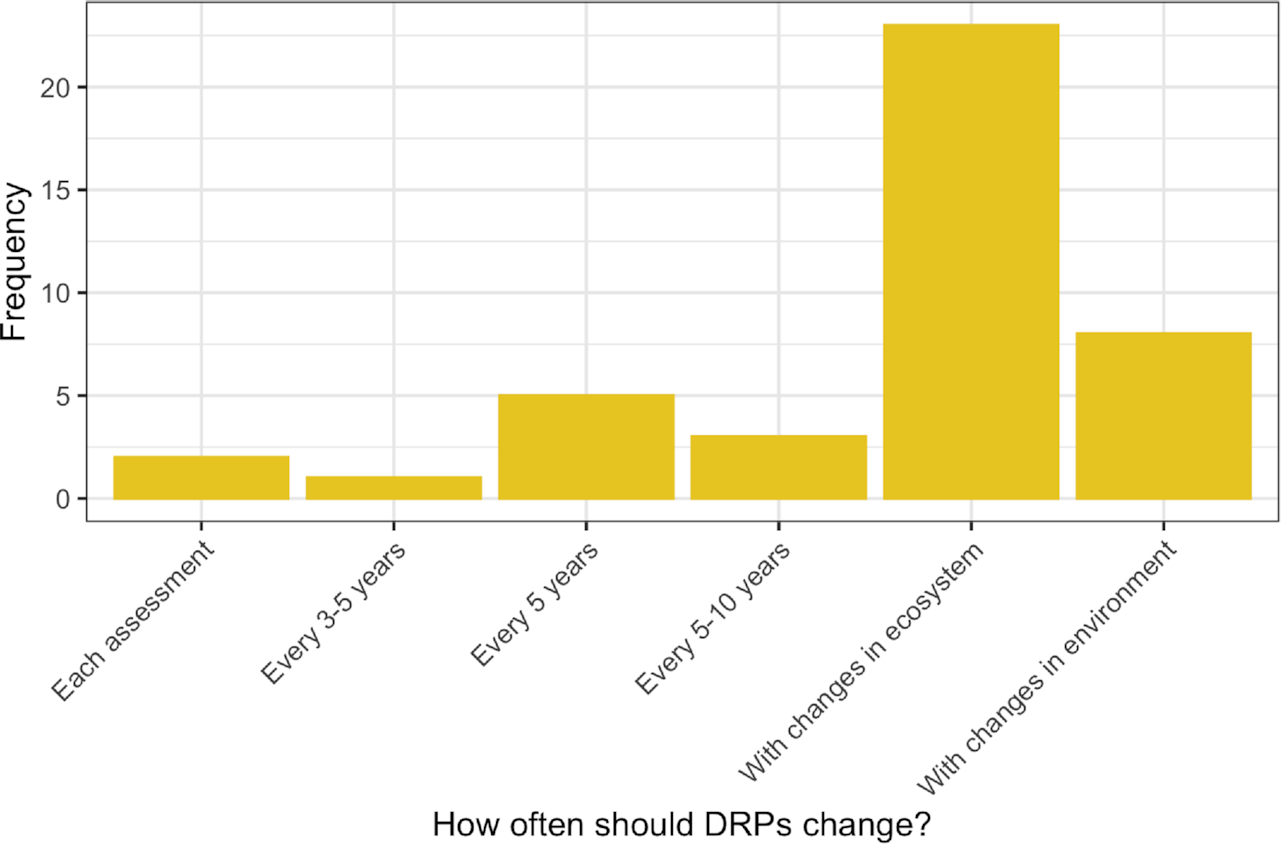
Implementation of dynamic reference points
Asked how often, in their experience, dynamic reference points had been implemented in fisheries management, 74% of respondents said never (n = 38), 16% said for very few fisheries (n = 8), and 10% said frequently (n = 5). When asked to identify barriers to implementation of dynamic reference points, institutional inertia and uncertainty about whether a change in productivity was lasting were the two most common responses (n = 13 each), followed by difficulty to operationalize and lack of consistent methods to apply them (n = 12 each; Fig. 5). We explored whether identified barriers were grouped according to respondent background but did not observe any clear patterns (Fig. 5). When asked for methods to address dynamic reference points, accounting for variable stock productivity was the most common answer (n = 9), followed by dynamic unfished biomass (B0; n = 7), ecosystem models (n = 5), time-varying natural mortality (n = 4), and regime-based methods (n = 3; Fig. 6). Fisheries and methods where they had been applied were dynamic B0 for scallop, tuna, harp (Pagophilus groenlandicus), and grey seals (Halichoerus grypus); variable natural mortality for cod; and time-varying spawning biomass per recruit for crab, menhaden, and groundfish. Methods were identified as useful: when there was a strong understanding of system dynamics (n = 7); when there were strong shifts in productivity (n = 5); in data rich systems (n = 3); and where climate drivers were important (n = 3). Methods were reported as limited: in data limited systems (n = 6) and when there was uncertainty about changes in productivity (n = 6) or system dynamics (n = 6). The data situations where these methods are applicable were in data rich systems (n = 18) and when it was possible to identify changes in productivity (n = 3). Additional survey response information can be found in Table 1.
Fig. 5.

Fig. 6.
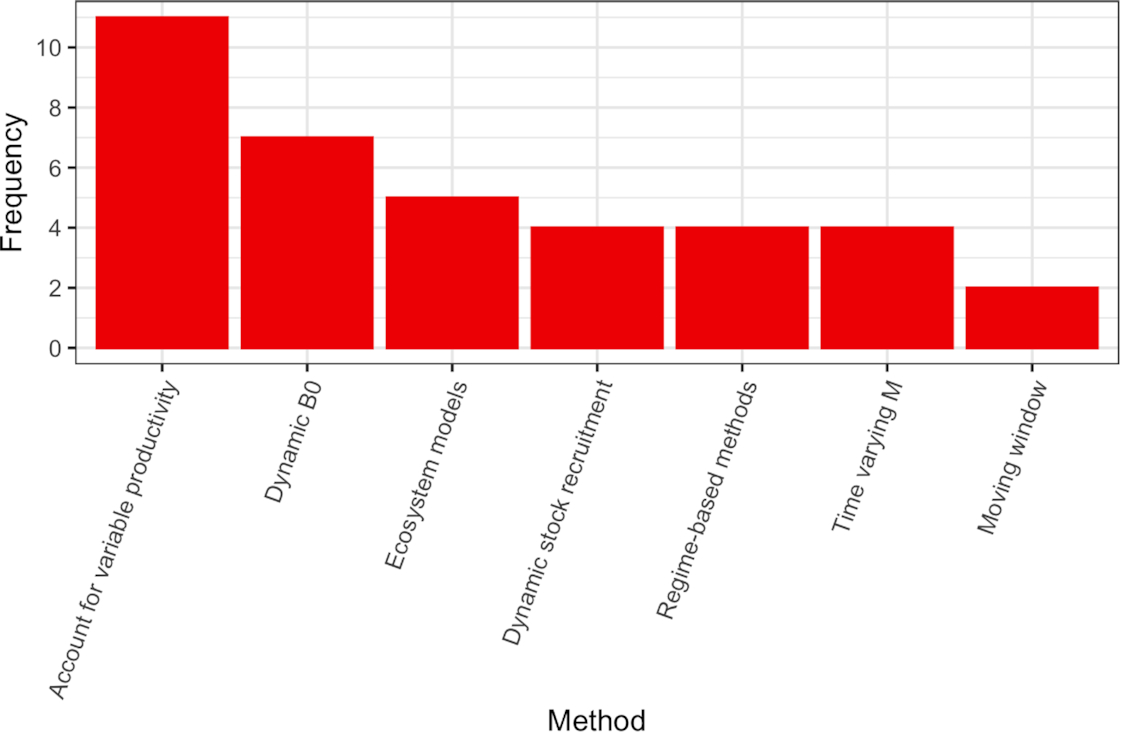
Discussion
The results of our survey indicated that there are a variety of scientific methods that have been used to implement dynamic reference points for fisheries management in many countries. The high proportion of respondents who indicated that changing ecosystem or fisheries productivity had been an issue in their experience (96%), and the low proportion who had observed any or frequent use of dynamic reference points (16% and 10%, respectively), suggests that there are barriers to their implementation. We acknowledge that some important fishing nations were not represented in our survey likely biasing our results, including Chile, Japan, Peru, and South Africa, that accounted for approximately 15% of global catches in 2018 (Pauly et al. 2020). However, most fishing nations that employ reference points were represented. We also acknowledge that tropical nations and fisheries were not well surveyed, indicating that our results are not representative of these regions and fisheries.
Institutional inertia and uncertainty whether a change was lasting were the two most frequently provided barriers. Institutional inertia can manifest through inertia in science (refusal of a new and improved assessment method, e.g., Berger et al. 2017) or in management (continual use of the same harvest strategy (i.e., Soomai 2017; Fulton 2021). There are trade-offs between management stability and management performance. Stability can be used as a metric of management performance, as it facilitates the implementation of management policies, maintains the credibility of management agencies, and allows for long-term planning by industry (Walters and Martell 2005). Institutional inertia that reduces decision space comes from both science and management, and initial scientific inertia may be compounded by managers or miscommunication between science and management (Arkema et al. 2006; Leslie and McLeod 2007). The scientific threshold of evidence for changing reference points within government departments may be high, and the resistance of the management system to allow large changes translates into a barrier of institutional inertia, resisting changes in reference points.
Survey results indicated that dynamic reference point approaches were only considered 10% of the time. This may be because dynamic reference point approaches are not often seen as necessary for good management of stocks or due to institutional and knowledge barriers to their implementation. Barriers preventing the integration of science into the decision-making process are not new, and decision-makers are more likely to include scientific research when it is considered relevant, credible (i.e., perceived by decision-makers as accurate, valid, and high quality), and legitimate (i.e., free from bias; Cash et al. 2002). These attributes are more likely to be achieved if collaborative and participatory approaches to knowledge exchange between scientists and decision-making have been applied (Cash et al. 2002, p. 20; Clarke et al. 2013).
Overcoming barriers to implementation of dynamic approaches to fisheries management can be achieved by creating a more responsive science-management process, through changes in data collection, stock assessment, and harvest control rule/catch advice formulation (Link et al. 2021). These measures may not be able to reduce uncertainty associated with if a change in productivity is lasting, which was tied for the most identified barrier; however, they may promote early detection of, and rapid response to, changes. For example, shifts in population productivity can be identified based on changes in population life-history characteristics (i.e., age at maturity, size at age for Atlantic cod and American plaice in Canada; Morgan et al. 2014). Ensuring that these data collection programs are in place can provide indicators to identify changing productivity. Additionally, stock assessments can be modified to include mechanisms that drive productivity (i.e., US west coast sablefish; Schirripa et al. 2009), parameters that vary over time (i.e., Atlantic cod, Canada; Minto et al. 2013; Zhang et al. 2020), or by using truncated time-series to estimate recruitment (i.e., Pacific salmon, herring, and pollock in Alaska; Rodionov 2004). Running single-species fisheries mortality targets in ecosystem models can provide strategic insight about whether ecosystem level thresholds will be exceeded, and if so, fishing mortality targets can be rescaled to ecosystem targets (Feco; i.e., Atlantic menhaden in the US and Atlantic cod, herring, whiting, Norway lobster, crabs, and scallops in the Irish Sea; Howell et al. 2021). Managers may also create a more responsive science-management process by modifying harvest control rules in response to changes in environmental variables or by modifying the allowable catch based on current estimates of stock or ecosystem productivity (i.e., Pacific groundfish stocks in Alaska; northern shrimp and snow crab in Canada; Holsman et al. 2020; Link et al. 2021; Marentette et al. 2021).
Survey results did not suggest a clear single approach to scientific methods or management actions for dynamic systems, with variability in responses about how often reference points should be changed, the methods that should be used to evaluate changes, how to set reference points, and what scenarios and data requirements are appropriate for such methods. Having a consistent approach may allow for easier implementation for new fisheries; however, the one-size fits all solution may lack the nuance required for fisheries with differing dynamics, data availability, and management structures. Species life history influences population dynamics and can provide advice about which approach may perform best (Berger 2019). Climate change adds the challenge of managing stocks that migrate across jurisdictional boundaries or are experiencing range shifts, whether they be management units within a country or across national borders (Pinsky et al. 2018; Palacios-Abrantes et al. 2021; Schuch et al. 2021). Addressing these issues could involve approaches that consider spatially resolved analyses (Berger et al. 2017).
Fish populations and ecosystems change through time, are presently being affected by climate change, and projections indicate exacerbated impacts as greenhouse gas emissions continue to increase (IPCC 2019). Fisheries management agencies are increasingly including environmental information in decision-making (Kulka 2022; Pepin et al. 2022). However, given the gap between the number of instances where changing ecosystem productivity was identified as a problem and the number of times that dynamic reference points were implemented, addressing barriers to implementation may be a way for management agencies to respond to highly dynamic ecosystems. There is no consensus on the scenario that warrants dynamic reference point use and how to apply them; however, there are now methods and case studies that we can look to when considering how to effectively manage fisheries in dynamic ecosystems.
Acknowledgements
We gratefully thank all survey respondents for sharing their knowledge and experiences with us. We thank an anonymous reviewer for constructive comments. TDE acknowledges funding from the Ocean Frontier Institute (OFI), Fisheries & Oceans Canada (DFO) Atlantic Fisheries Fund (AFF), and the Canadian Natural Sciences and Engineering Research Council (NSERC) Discovery Grant. RR and CAS were supported by Memorial University School of Graduate Studies baseline funding. MDR was supported by a Vanier Canada Graduate Scholarship. Open access of this article was supported by the Memorial University Open Access Author Fund.
References
A’mar Z.T., Punt A.E., Dorn M.W. 2009. The evaluation of two management strategies for the Gulf of Alaska walleye pollock fishery under climate change. ICES Journal of Marine Science, 66(7): 1614–1632.
Arkema K.K., Abramson S.C., Dewsbury B.M. 2006. Marine ecosystem-based management: from characterization to implementation. Frontiers in Ecology and the Environment 4(10): 525–532.
Bakun A., Babcock E.A., Lluch-Cota S.E., Santora C., Salvadeo C.J. 2010. Issues of ecosystem-based management of forage fisheries in “open” non-stationary ecosystems: the example of the sardine fishery in the Gulf of California. Reviews in Fish Biology and Fisheries, 20(1): 9–29.
Berger A.M. 2019. Character of temporal variability in stock productivity influences the utility of dynamic reference points. Fisheries Research, 217: 185–197.
Berger A.M., Goethel D.R., Lynch P.D., Quinn T., Mormede S., McKenzie J., Dunn A. 2017. Space oddity: the mission for spatial integration. Canadian Journal of Fisheries and Aquatic Sciences, 74(11): 1698–1716.
Caddy J.F., Mahon R. 1995. Reference points for fisheries management. Food and Agriculture Organization of the United Nations Rome.
Carpenter S.R., Brock W.A., Hansen G.J.A., Hansen J.F., Hennessy J.M., Isermann D.A., et al. 2017. Defining a safe operating space for inland recreational fisheries. Fish and Fisheries, 18(6): 1150–1160.
Cash D., Clark W.C., Alcock F., Dickson N.M., Eckley N., Jäger J. 2002. Salience, credibility, legitimacy and boundaries: linking research, assessment and decision making.
Clarke B., Stocker L., Coffey B., Leith P., Harvey N., Baldwin C., et al. 2013. Enhancing the knowledge–governance interface: coasts, climate and collaboration. Ocean & coastal management, 86: 88–99.
Collie J.S., Bell R.J., Collie S.B., Minto C. 2021. Harvest strategies for climate-resilient fisheries. ICES Journal of Marine Science, 78(8): 2774–2783.
Dempsey D.P., Gentleman W.C., Pepin P., Koen-Alonso M. 2018. Explanatory power of human and environmental pressures on the fish community of the Grand Bank before and after the biomass collapse. Frontiers in Marine Science, 5: 37.
Fulton E.A. 2021. Opportunities to improve ecosystem-based fisheries management by recognizing and overcoming path dependency and cognitive bias. Fish and Fisheries, 22(2): 428–448.
Haltuch M.A., Punt A.E. 2011. The promises and pitfalls of including decadal-scale climate forcing of recruitment in groundfish stock assessment. Canadian Journal of Fisheries and Aquatic Sciences, 68(5): 912–926.
Haltuch M.A., Punt A.E., Dorn M.W. 2009. Evaluating the estimation of fishery management reference points in a variable environment. Fisheries Research, 100(1): 42–56.
Hilborn R., Walters C.J. 1992. Quantitative fisheries stock assessment: choice, dynamics and uncertainty. Springer Science & Business Media.
Hill K.T., Crone P.R., Zwolinski J.P. 2019. Assessment of the Pacific sardine resource in 2019 for US management in 2019–20.
Holsman K.K., Haynie A.C., Hollowed A.B., Reum J.C.P., Aydin K., Hermann A.J., et al. 2020. Ecosystem-based fisheries management forestalls climate-driven collapse. Nature Communications, 11(1): 4579.
Holt C.A., Michielsens C.G. 2020. Impact of time-varying productivity on estimated stock—recruitment parameters and biological reference points. Canadian Journal of Fisheries and Aquatic Sciences, 77(5): 836–847.
Howell D., Schueller A.M., Bentley J.W., Buchheister A., Chagaris D., Cieri M., et al. 2021. Combining ecosystem and single-species modeling to provide ecosystem-based fisheries management advice within current management systems. Frontiers in Marine Science, 7: 1163.
IPCC. 2019. Special report on the ocean and cryosphere in a changing climate. Edited by H.-O. Pörtner, D.C. Roberts, V. Masson-Delmotte, P. Zhai, M. Tignor, E. Poloczanska, K. Mintenbeck, A. Alegría, M. Nicolai, A. Okem, J. Petzold, B. Rama, N.M. Weyer. Available from https://www.ipcc.ch/srocc/cite-report/ [accessed 23 June 2021].
Kasperski S., DePiper G.S., Haynie A.C., Blake S., Colburn L.L., Freitag A., et al. 2021. Assessing the state of coupled social-ecological modeling in support of ecosystem based fisheries management in the United States. Frontiers in Marine Science, 8: 181.
King J.R., McFarlane G.A., Punt A.E. 2015. Shifts in fisheries management: adapting to regime shifts. Philos. Trans. R. Soc. B Biol. Sci. 370(1659): 20130277.
Klaer N.L., O’Boyle R.N., Deroba J.J., Wayte S.E., Little L.R., Alade L.A., Rago P.J. 2015. How much evidence is required for acceptance of productivity regime shifts in fish stock assessments: are we letting managers off the hook? Fisheries Research, 168: 49–55.
Kulka D.W. 2022. An accounting of integration of environmental variables in fishery stock assessments in Canada. Fisheries and Oceans Canada, Northwest Atlantic Fisheries Centre, St. John’s, NL.
Leslie H.M., McLeod K.L. 2007. Confronting the challenges of implementing marine ecosystem-based management. Frontiers in Ecology and the Environment, 5(10): 540–548.
Lindegren M., Möllmann C., Nielsen A., Stenseth N.C. 2009. Preventing the collapse of the Baltic cod stock through an ecosystem-based management approach. Proceedings of the National Academy of Sciences of the United States of America, 106(34): 14722–14727.
Link J.S., Marshak A.R. 2021. Ecosystem-based fisheries management: progress, importance, and impacts in the United States. Oxford University Press, Oxford.
Link J.S., Karp M.A., Lynch P., Morrison W.E., Peterson J. 2021. Proposed business rules to incorporate climate-induced changes in fisheries management. ICES Journal of Marine Science, 78(10): 3562–3580.
MacCall A., Klingbeil R., Methot R. 1985. Recent increased abundance and potential productivity of pacific mackerel. CalCOFI Report 26.
Marentette J.R., Kronlund A.R., Cogliati K.M. 2021. Specification of precautionary approach reference points and harvest control rules in domestically managed and assessed key harvested stocks in Canada. Canadian Science Advisory Secretariat (CSAS).
Minto C., Mills Flemming J., Britten G.L., Worm B. 2013. Productivity dynamics of Atlantic cod. Canadian Journal of Fisheries and Aquatic Sciences, 71: 203–216.
Morgan M.J., Shelton P.A., Rideout R.M. 2014. Varying components of productivity and their impact on fishing mortality reference points for Grand Bank Atlantic cod and American plaice. Fisheries Research, 155: 64–73.
O’Leary C.A., Thorson J.T., Miller T.J., Nye J.A. 2020. Comparison of multiple approaches to calculate time-varying biological reference points in climate-linked population-dynamics models.ICES Journal of Marine Science, 77(3): 930–941.
Palacios-Abrantes J., Frölicher T.L., Reygondeau G., Sumaila U.R., Tagliabue A., Wabnitz C.C., Cheung W.W. 2021. Timing and magnitude of climate-driven range shifts in transboundary fish stocks challenge their management. Global Change Biology, 28: 2312–2326.
Pauly, D., Zeller, D., Palomares, M.L.D. (eds) 2020. Sea Around Us Concepts, Design and Data. Available from https://www.seaaroundus.org/.
Pedersen M.W., Berg C.W. 2017. A stochastic surplus production model in continuous time. Fish and Fisheries, 18(2): 226–243.
Penrod J., Preston D.B., Cain R.E., Starks M.T. 2003. A discussion of chain referral as a method of sampling hard-to-reach populations. Journal of Transcultural Nursing, 14(2): 100–107.
Pepin P., King J., Holt C., Smith H., Shackell N., Hedges K., Bundy A. 2022. Incorporating knowledge of changes in climatic, oceanographic and ecological conditions in Canadian stock assessments. Fish and Fisheries, 23(6): 1332–1346.
Peterman R.M., Pyper B.J., Grout J.A. 2000. Comparison of parameter estimation methods for detecting climate-induced changes in productivity of Pacific salmon (Oncorhynchus spp.). Canadian Journal of Fisheries and Aquatic Sciences, 57(1): 181–191.
Peterman R.M., Pyper B.J., MacGregor B.W. 2003. Use of the Kalman filter to reconstruct historical trends in productivity of Bristol Bay sockeye salmon (Oncorhynchus nerka). Canadian Journal of Fisheries and Aquatic Sciences, 60(7): 809–824.
Pinsky M.L., Reygondeau G., Caddell R., Palacios-Abrantes J., Spijkers J., Cheung W.W.L. 2018. Preparing ocean governance for species on the move. Science, 360(6394): 1189–1191.
Punt A.E., A’mar T., Bond N.A., Butterworth D.S., de Moor C.L., De Oliveira J.A.A., et al. 2014. Fisheries management under climate and environmental uncertainty: control rules and performance simulation. ICES Journal of Marine Science, 71(8): 2208–2220.
Punt A.E., Castillo-Jordán C., Hamel O.S., Cope J.M., Maunder M.N., Ianelli J.N. 2021. Consequences of error in natural mortality and its estimation in stock assessment models. Fisheries Research, 233: 105759.
Rodionov S., Overland J.E. 2005. Application of a sequential regime shift detection method to the Bering Sea ecosystem. ICES Journal of Marine Science, 62(3): 328–332.
Rodionov S.N. 2004. A sequential algorithm for testing climate regime shifts. Geophysical Research Letters, 31(9).
Schirripa M.J., Goodyear C.P., Methot R.M. 2009. Testing different methods of incorporating climate data into the assessment of US West Coast sablefish. ICES Journal of Marine Science, 66(7): 1605–1613.
Schuch E., Gabbert S., Richter A.P. 2021. Institutional inertia in European fisheries—insights from the Atlantic horse mackerel case. Marine Policy, 128: 104464.
Soomai S.S. 2017. The science–policy interface in fisheries management: insights about the influence of organizational structure and culture on information pathways. Marine Policy, 81: 53–63.
Spence M.A., Dolder P.J., Nash R., Thorpe R.B. 2021. The use of a length-structured multispecies model fitted directly to data in near-real time as a viable tool for advice. Frontiers in Marine Science, 1373.
Szuwalski C. 2013. Production is a poor metric for identifying regime-like behavior in marine stocks. Proceedings of the National Academic Sciences of the United States of America, 110(16).
Szuwalski C.S., Hollowed A.B. 2016. Climate change and non-stationary population processes in fisheries management. ICES Journal of Marine Science, 73(5): 1297–1305.
Tang X., Zheng N., Rideout R.M., Wang S., Zhang F. 2021. Identification of recruitment regime shifts with a hidden Markov stock-recruitment model. ICES Journal of Marine Science, 78(7): 2591–2602.
Townsend H., Harvey C.J., deReynier Y., Davis D., Zador S.G., Gaichas S., et al. 2019. Progress on implementing ecosystem-based fisheries management in the United States through the use of ecosystem models and analysis. Frontiers in Marine Science, 6: 641. Frontiers.
Travis J., Coleman F.C., Auster P.J., Cury P.M., Estes J.A., Orensanz J., et al. 2014. Integrating the invisible fabric of nature into fisheries management. Proceedings of the National Academy of Sciences of the United Sciences of America, 111(2): 581–584.
van Deurs M., Brooks M.E., Lindegren M., Henriksen O., Rindorf A. 2021. Biomass limit reference points are sensitive to estimation method, time-series length and stock development. Fish and Fisheries, 22(1): 18–30.
Vert-pre K.A., Amoroso R.O., Jensen O.P., Hilborn R. 2013a. Frequency and intensity of productivity regime shifts in marine fish stocks. Proceedings of the National Academy of Sciences of the United States of America, 110(5): 1779–1784.
Vert-pre K.A., Amoroso R.O., Jensen O.P., Hilborn R. 2013b. Reply to Szuwalski: policies robust to uncertainty in causes of productivity changes are needed. Proceedings of the National Academy of Sciences of the United States of America, 110(16).
Walters C.J., Martell S.J. 2005. Fisheries ecology and management. Princeton University Press.
Worm B., Hilborn R., Baum J.K., Branch T.A., Collie J.S., Costello C., et al. 2009. Rebuilding global fisheries. Science, 325(5940): 578–585.
Zhang F., Eddy T., Duplisea D., Robertson M., Ruiz R., Solberg C. 2021. Report on Ocean Frontier Institute Workshop on fisheries management reference points in highly dynamic ecosystems.
Zhang F., Reid K.B., Nudds T.D. 2018. Ecosystem change and decadal variation in stock–recruitment relationships of Lake Erie yellow perch (Perca flavescens). ICES Journal of Marine Science, 75(2): 531–540.
Zhang F., Rideout R.M., Cadigan N.G. 2020. Spatiotemporal variations in juvenile mortality and cohort strength of Atlantic cod (Gadus morhua) off Newfoundland and Labrador. Canadian Journal of Fisheries and Aquatic Sciences, 77(3): 625–635.
Information & Authors
Information
Published In
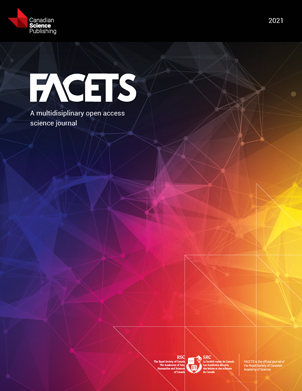
FACETS
Volume 8 • 2023
Pages: 1 - 10
Editor: S.J. Cooke
History
Received: 12 October 2022
Accepted: 6 March 2023
Version of record online: 10 July 2023
Copyright
© 2023 Authors Eddy, Robertson, Ruiz-Díaz, Solberg, and Zhang; and The Crown. This work is licensed under a Creative Commons Attribution 4.0 International License (CC BY 4.0), which permits unrestricted use, distribution, and reproduction in any medium, provided the original author(s) and source are credited.
Data Availability Statement
Survey response data are not available due to human ethics confidentiality requirements.
Key Words
Sections
Subjects
Plain Language Summary
Fisheries science and management to account for shifting baselines in dynamic ecosystems
Authors
Author Contributions
Conceptualization: TDE
Data curation: TDE
Formal analysis: TDE
Funding acquisition: TDE
Methodology: TDE, DD, FZ
Visualization: TDE
Writing – original draft: TDE
Writing – review & editing: TDE, DD, MDR, RR, CAS, FZ
Competing Interests
The authors have no competing interests to disclose.
Metrics & Citations
Metrics
Other Metrics
Citations
Cite As
Tyler D. Eddy, Daniel Duplisea, Matthew D. Robertson, Raquel Ruiz-Díaz, C. Abraham Solberg, and Fan Zhang. 2023. Barriers to implementation of dynamic reference points in fisheries management. FACETS.
8: 1-10.
https://doi.org/10.1139/facets-2022-0216
Export Citations
If you have the appropriate software installed, you can download article citation data to the citation manager of your choice. Simply select your manager software from the list below and click Download.
Cited by
1. An Advanced Logic to Optimize the Product Search Engines Based on Dynamic Facet Ordering Model to Support E-Commerce Industry
2. Linking crustacean life history to fishery management controls and reference points